Smart Civic Tech @ Bloustein
The Bloustein School is pursuing a range of ongoing initiatives related to the adoption of smart civic technology and the use of data for public good. The 21st century has presented new challenges along with new opportunities to use data to solve grand public challenges. Leveraging data presents new opportunities to encourage civic engagement through cross-disciplinary collaboration and the implementation of cutting-edge technologies.
Minds and Machines
Intelligent tools are changing the world, our lives, and our work in expected and unexpected ways. They have led to advancements in our health, livelihood, and overall quality of life. At the same time, their misuse can have harmful individual and societal outcomes, such as loss of privacy, algorithmic bias, group marginalization, and the propagation of misinformation. Today’s interconnected world requires intelligence in science and technology to simultaneously support growing needs and safeguard us from unintended consequences.
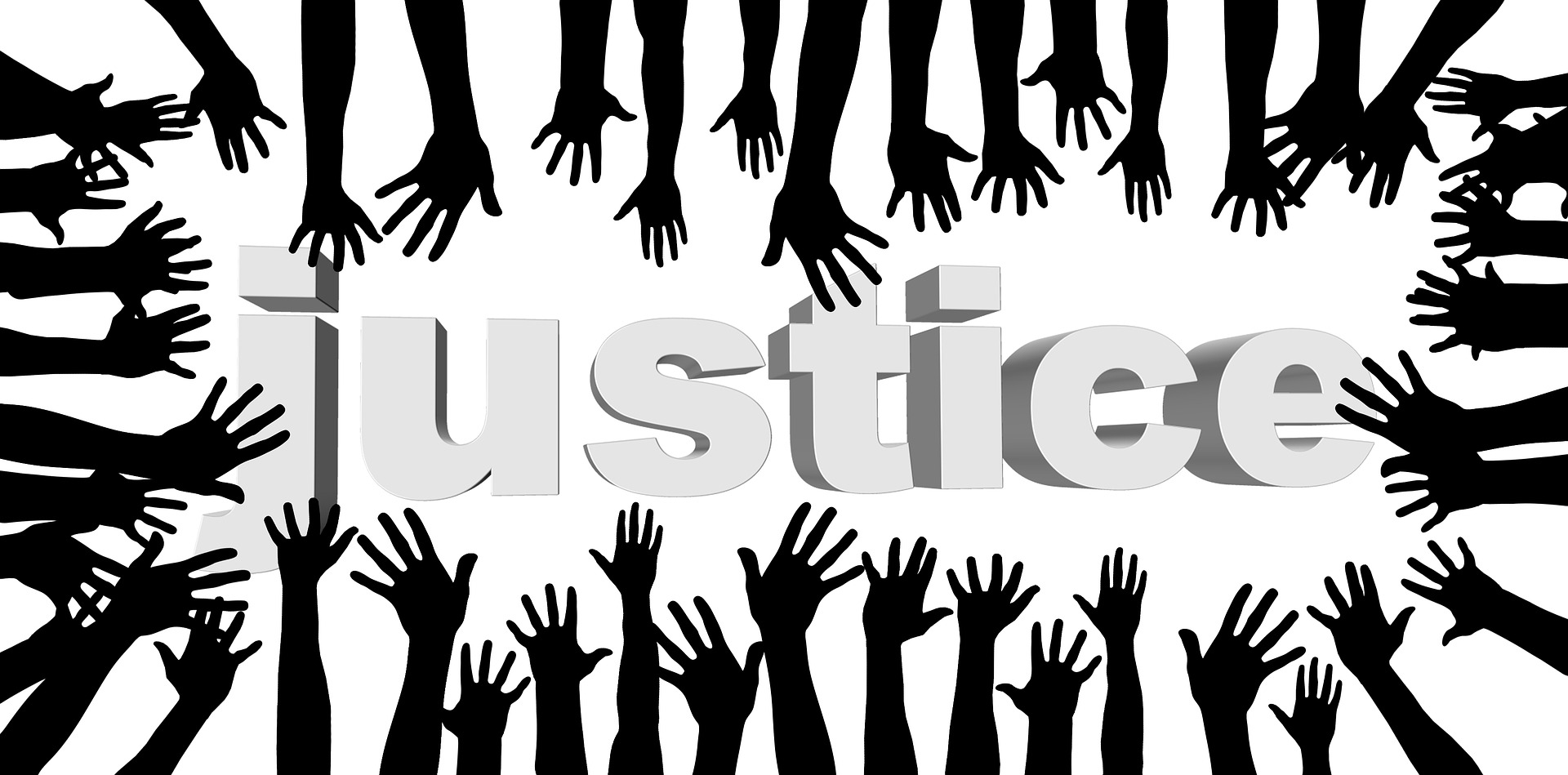
Smart Civic Technology and Social Justice Cluster
The Smart Civic Technology and Social Justice Cluster is an interdisciplinary team of faculty and staff researchers who are committed to deploying civic technology with a focus on responsibly using technology to improve social justice and equity in communities.
Tracking COVID-19’s Impact on New Jersey With Anonymized Cell Phone Location Data
Using data from anonymized mobile devices and building footprints, we examined how mobility patterns changed in NJ for the period of March 1, 2020, to May 17, 2020. This two and half month span includes the period with the maximum restrictions on individuals and businesses.
An interactive dashboard was built based upon this work allowing comparisons between 2019 and 2020 movement patterns and visits to points of interest in all 21 counties of New Jersey.
The Rutgers Urban & Civic Informatics Laboratory is taking this work further with additional ongoing projects and initiatives to leverage new and emerging technologies for the public good.
Researcher: Piyushimita (Vonu) Thakuriah, Ph.D.
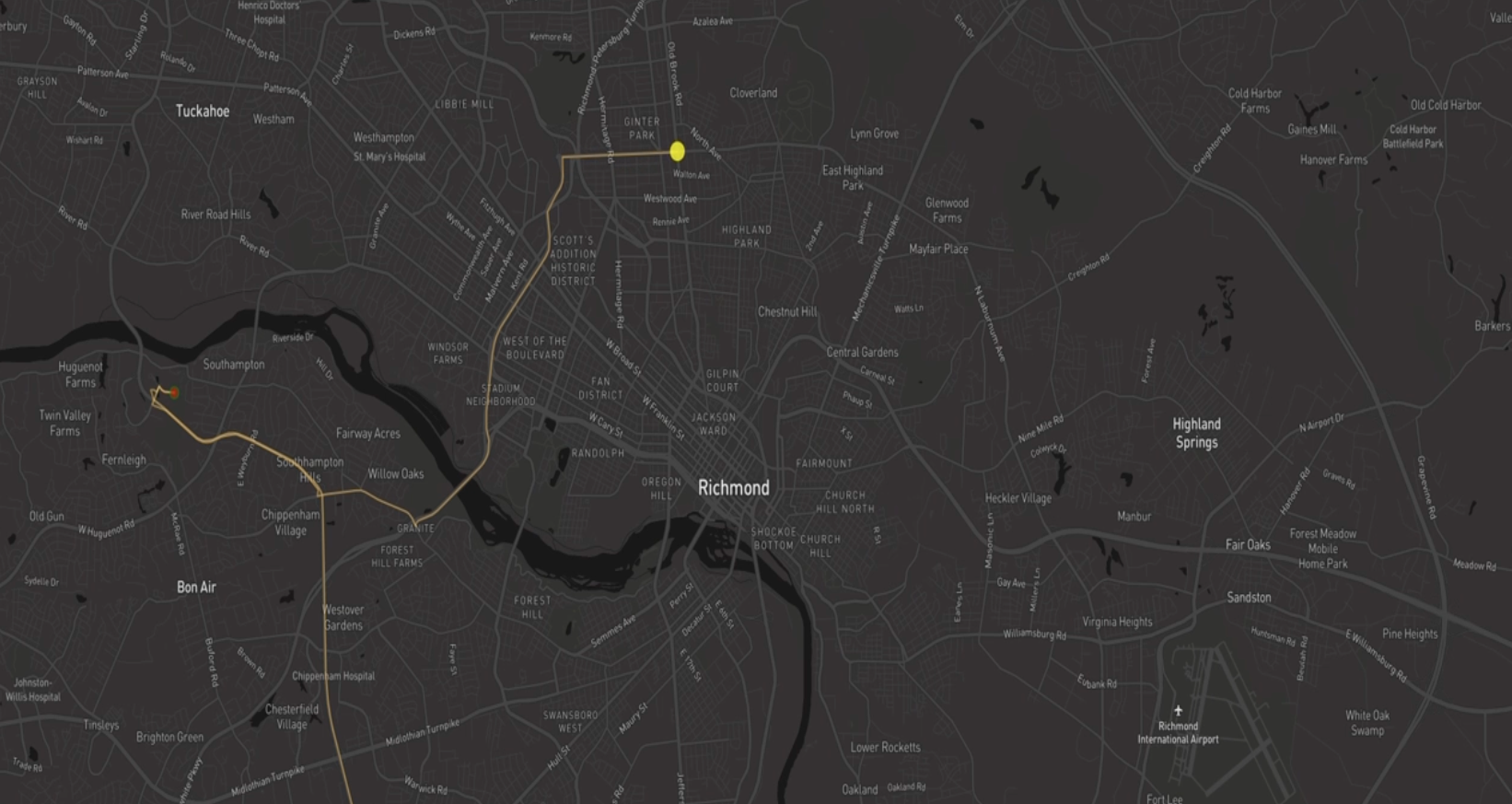
Machine learning neighborhood-level preferences for automated vehicles
Automated Vehicles (AVs) have gained substantial attention in recent years as the technology has matured. Researchers and policymakers envision that AV deployment will change transportation, development patterns, and other urban systems. Researchers have examined AVs and their potential impacts with two methods: (1) survey-based studies of AV preferences and (2) simulation-based estimation of secondary impacts of varied AV deployment strategies, such as Shared AVs (SAVs) and Privately-owned AVs (PAVs).
Researcher: Wenwen Zhang, Ph.D.

Future of Work in the Age of Intelligent Machines
The Bloustein School currently serves as the host and manager for the State of New Jersey’s multi-agency integrated longitudinal data system. The New Jersey Education to Earnings Data System (NJEEDS), which resides at the John J. Heldrich Center for Workforce Development, is a centralized longitudinal data system for education and workforce data. Its mission is to create a single place for evidenced-based policymaking by combining state education, post-secondary education, employment, and workforce longitudinal data to improve governance efforts, policymaking, and the performance of educational, and workforce initiatives.
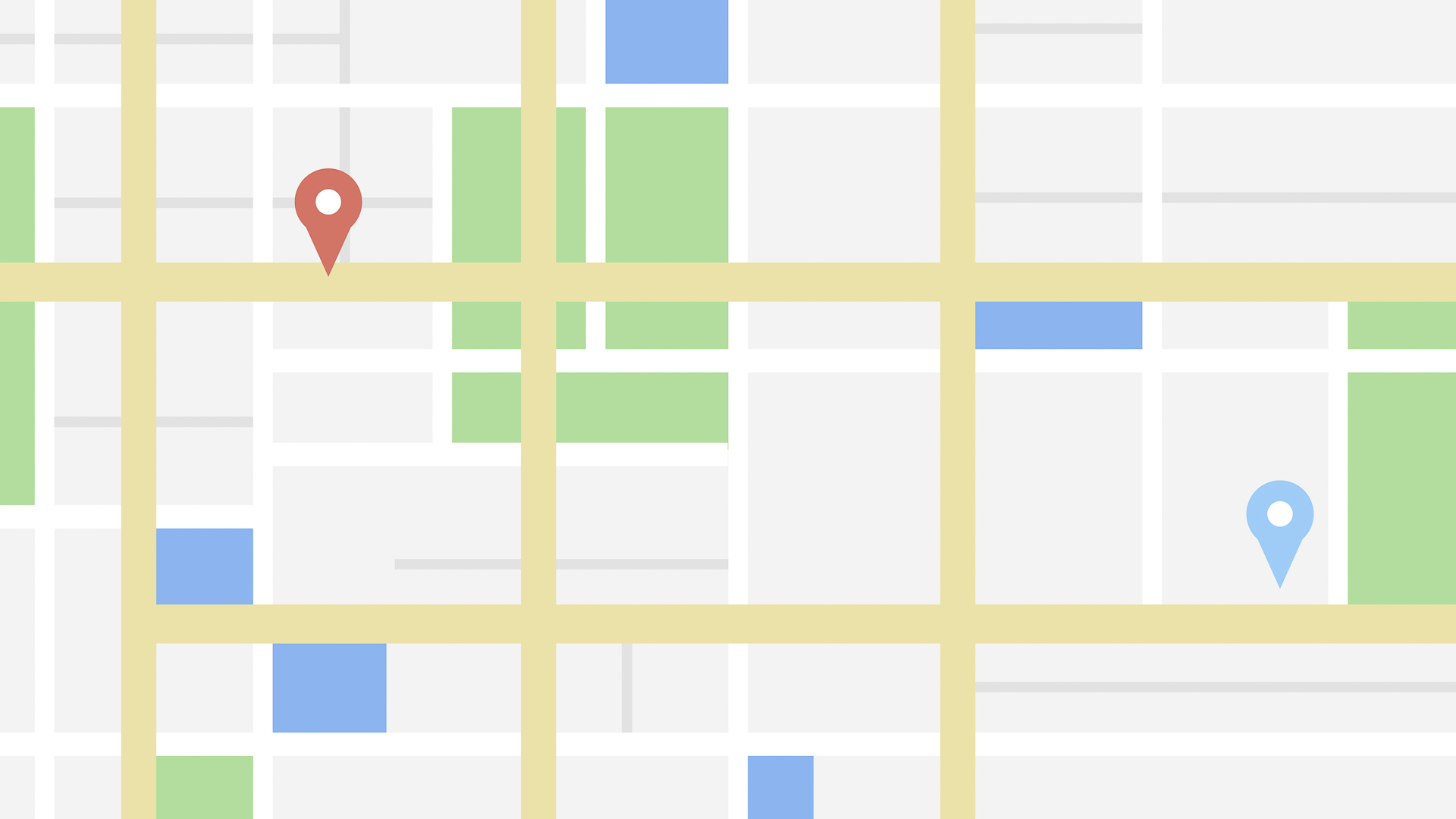
Intensive and Extensive Approaches to Crowdsourcing Spatial Data
In this project, a combination of key informant interviews and analysis of financial statements, company job posts, and marketing materials to explore how two major location-based services (LBS) in the United States, Yelp and Google Maps, leverage volunteer top contributor programs to ensure access to reliable spatial data. Through the Elite Squad program, paid Yelp staff take an active curatorial role growing the company’s volunteer reviewer base in select urban regions in North America, with a focus on urban regions with high median incomes and education levels. Google’s Local Guides program, on the other hand, uses an extensive, self-service model to collect data on a global scale. Both companies enroll and motivate users in ways that present unpaid review labor as affirming, with emphases that reflect their scalar strategies: Yelp stressing tight-knit sociality and Google global altruism.
Researcher: Will Payne, Ph.D.
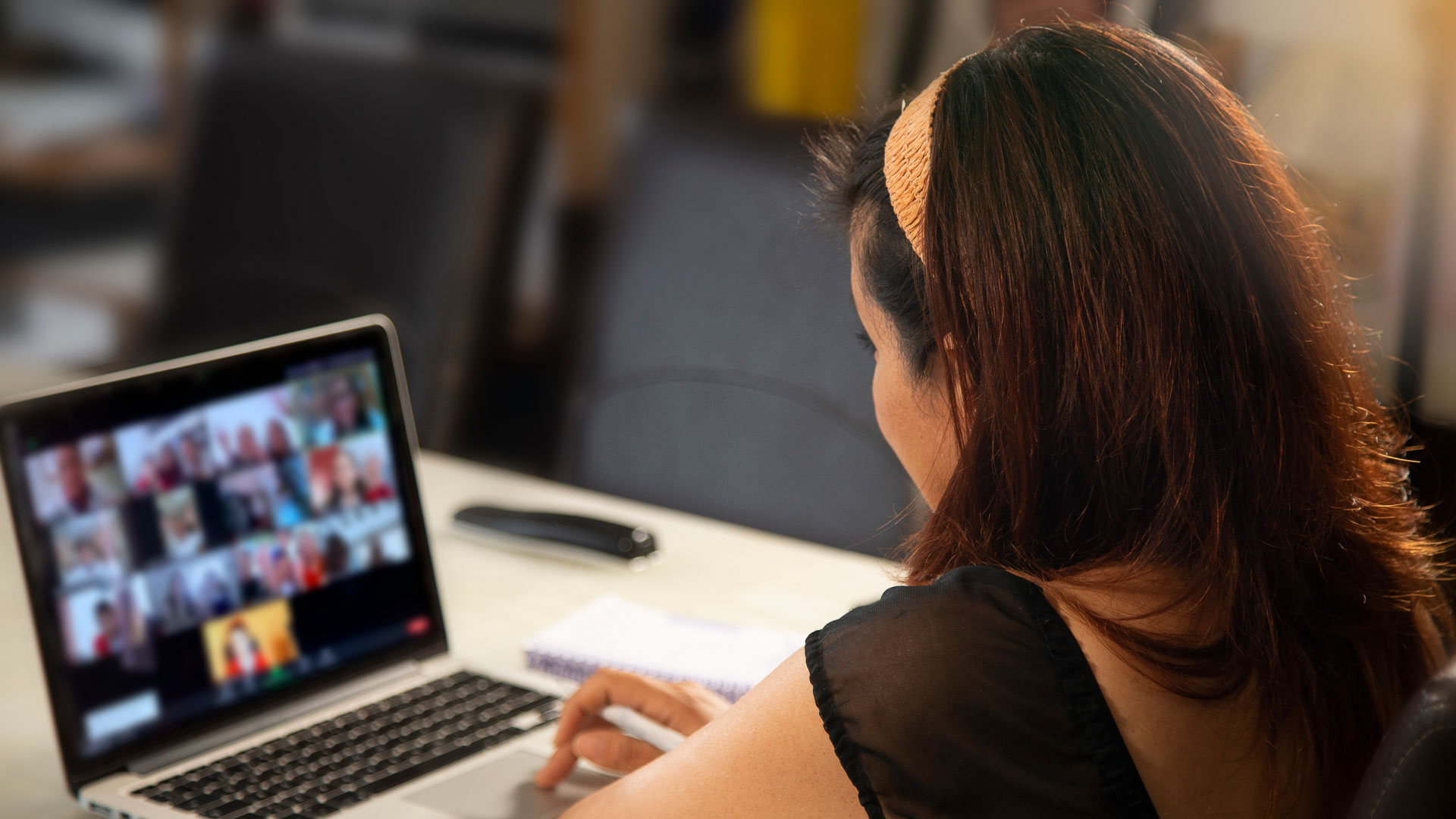
Remote Workers Don’t Escape the Power Structure
Even before the COVID pandemic, remote workers were learning that physical untethering does not mean freedom from corporate power relationships. Remote workers often use smart work spaces that are created through access to wireless networks and mobile cloud computing collaboration software. Yet the power relations embedded in these overlapping physical and cyberspaces function to control the spatial and temporal fragmentation of related work activities.
Researcher: Clinton J. Andrews, Ph.D.